Cracking the Code: Unveiling the Secrets of Dissertation Data Analysis
Joe Howard -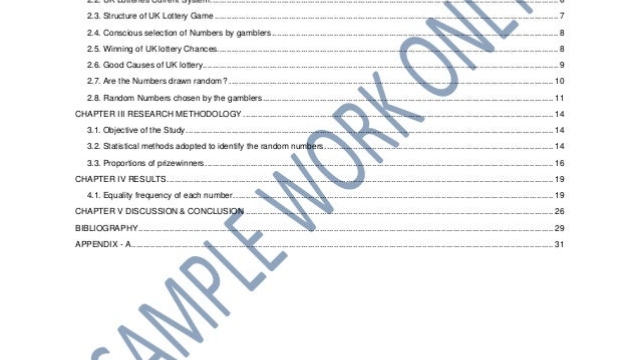
Dissertations and capstones mark the culmination of a long and arduous academic journey for many students. These comprehensive research projects involve a multitude of components, one of the most critical being data analysis. Unlocking the secrets of dissertation data analysis is no easy feat, but with the right tools and guidance, this process can become a well-defined and manageable task.
Data analysis forms the backbone of any dissertation project, as it involves the systematic examination, interpretation, and presentation of data collected during the research process. It plays a vital role in drawing meaningful conclusions, identifying trends, and establishing the significance of the study’s findings. However, the complexity and vastness of data can often appear daunting, leaving many students confused about where to start or how to make sense of the figures and statistics staring back at them.
In this article, we will delve into the intricacies of dissertation data analysis, revealing the key steps and strategies that will help you crack the code and unravel its secrets. We will explore various approaches to data analysis, highlighting both quantitative and qualitative methods, as well as the tools and software available to assist you in your analytical journey. By the end of this article, you will have gained a clearer understanding of how to navigate through the intricacies of dissertation data analysis and harness its power to bolster the impact of your research. So, let’s embark on this enlightening expedition together and unlock the hidden gems that lie within your data.
Understanding Dissertation Data Analysis
Data analysis is a critical component of dissertations and capstones. It involves the systematic examination and interpretation of data collected during the research process. By analyzing this data, researchers can gain insights, draw conclusions, and make meaningful contributions in their areas of study.
In the realm of dissertations, data analysis serves as a crucial step in the research journey. It allows researchers to organize, process, and make sense of the vast amount of information gathered. Through data analysis, researchers can identify patterns, relationships, and trends, which can help support or refute their research hypotheses.
Dissertation data analysis is not a one-size-fits-all approach. Depending on the nature of the research and the type of data collected, different methods and techniques may be employed. From qualitative analysis to quantitative analysis, researchers have a range of options to choose from to suit their study objectives and research questions.
Overall, dissertation data analysis is a vital aspect of research as it enables researchers to derive meaningful insights from their data. By applying appropriate analysis techniques, researchers can uncover valuable information that contributes to the existing knowledge and paves the way for further research in their respective fields.
Choosing the Right Analytical Approaches
When it comes to conducting effective dissertation data analysis, it is crucial to select the right analytical approaches that align with your research goals and data characteristics. By choosing the most suitable methods, you can uncover valuable insights and draw meaningful conclusions from the data. In this section, we will discuss three important factors to consider when making this decision.
Firstly, the nature of your research question plays a significant role in determining the appropriate analytical approach. Are you aiming to explore relationships between variables, compare groups, or make predictions? Understanding the specific objective of your study will guide you in selecting the most relevant methods, such as correlation analysis, t-tests, or regression analysis.
Secondly, consider the type of data you have collected for your dissertation or capstone. Is it qualitative or quantitative? Qualitative data often requires different analytical approaches, such as thematic analysis or content analysis, compared to quantitative data that may involve statistical techniques like descriptive statistics or inferential analysis. By identifying the nature of your data, you can identify the appropriate analytical methods to apply.
Lastly, take into account the scope and size of your dataset. Are you working with a small, manageable sample size or a large, complex dataset? Analytical approaches such as factor analysis or cluster analysis may be more suitable for dealing with larger datasets, while methods like chi-square tests or simple regression may be better suited for smaller, focused samples.
In summary, choosing the right analytical approaches for your dissertation data analysis requires consideration of your research question, the type of data you have collected, and the scope of your dataset. By carefully evaluating these factors, you can ensure that your analysis is meaningful, accurate, and aligned with your research goals.
Interpreting and Presenting the Findings
In the process of dissertation data analysis, the findings obtained are a crucial component that requires interpretation and effective presentation. This section delves into the significance of interpreting and presenting the findings, highlighting the key practices that can enhance the understanding and impact of the research outcomes.
Understanding the findings is the first step in interpreting the data analysis results. This involves examining the patterns, trends, and relationships uncovered from the collected data. By critically analyzing the findings, researchers can identify key insights, draw conclusions, and establish connections between variables or concepts. A thorough understanding of the findings is essential for effectively communicating the implications and significance of the research.
Once the findings have been interpreted, it is crucial to present them in a clear and concise manner. Effective presentation of the findings ensures that the research outcomes are accessible and understandable to the intended audience. Presentation methods can vary depending on the nature of the data and the research objectives. It is important to utilize appropriate visual aids such as graphs, charts, and tables to enhance the visualization and comprehension of the findings. Well-designed visual representations can facilitate the communication of complex information and convey the main messages derived from the data analysis.
Furthermore, the presentation of findings should not only focus on the results but also provide context and explanation. Researchers should consider the broader theoretical and practical implications of their findings and provide a comprehensive analysis that goes beyond descriptive statistics. By offering interpretations and insights into the significance of the results, the audience gains a deeper understanding of the research’s contribution to the field.
In conclusion, the interpretation and presentation of findings play a vital role in dissertation data analysis. Through careful interpretation, researchers can draw meaningful conclusions from their data, while effective presentation ensures that these findings are accessible and impactful. By following best practices and using appropriate visualization techniques, researchers can effectively communicate their research outcomes to a wider audience, contributing to the advancement of scholarly knowledge and understanding.
Archives
- March 2024
- February 2024
- January 2024
- December 2023
- November 2023
- October 2023
- September 2023
- August 2023
- July 2023
- June 2023
- May 2023
- April 2023
- March 2023
- February 2023
- January 2023
- December 2022
- November 2022
- October 2022
- September 2022
- August 2022
- July 2022
- June 2022
- May 2022
- April 2022
- March 2022
- February 2022
- January 2022
Calendar
M | T | W | T | F | S | S |
---|---|---|---|---|---|---|
1 | 2 | 3 | 4 | 5 | 6 | 7 |
8 | 9 | 10 | 11 | 12 | 13 | 14 |
15 | 16 | 17 | 18 | 19 | 20 | 21 |
22 | 23 | 24 | 25 | 26 | 27 | 28 |
29 | 30 | 31 |