Cracking the Code: Mastering Dissertation Data Analysis
Joe Howard -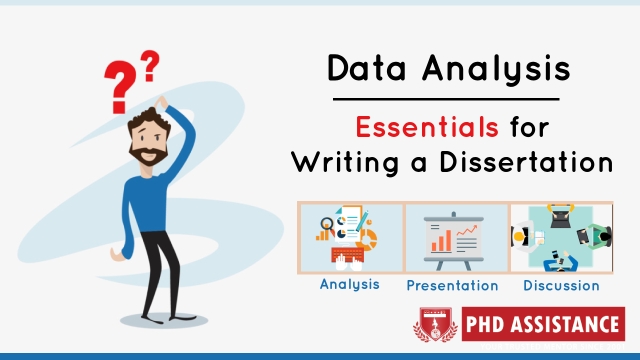
Are you ready to unlock the secrets hidden within dissertations and capstones? As aspiring scholars, we dedicate countless hours to conducting research, collecting data, and organizing our thoughts into comprehensive documents. But the journey to academic excellence doesn’t end there. Once all the data is gathered, we are faced with the exhilarating yet daunting task of dissertation data analysis.
Dissertation data analysis is a vital step in the research process, where we dive deep into the collected data to uncover meaningful patterns, draw accurate conclusions, and provide insights that contribute to the body of knowledge in our respective fields. It is through this analytical exploration that we can truly give life to our research and validate our findings.
Understanding the intricacies of dissertation data analysis is no small feat. It requires a combination of technical skills, critical thinking, and a keen eye for detail. From choosing the most appropriate statistical methods to interpreting the results, each step along the way holds the key to unlocking the true potential of our research.
In this article, we will delve into the world of dissertation data analysis, exploring different methodologies, tools, and techniques that can help you master this crucial aspect of your academic journey. Whether you’re a seasoned researcher or just starting out, this guide will equip you with the knowledge and skills necessary to crack the code of dissertation data analysis and elevate the impact of your research to new heights. So, let’s embark on this transformative journey together and unravel the mysteries of deciphering data for dissertations and capstones.
Understanding Dissertation Data Analysis
Data analysis is a crucial component of dissertations and capstones. It involves the systematic examination and interpretation of data to derive meaningful insights and conclusions. By employing various statistical techniques and analytical methods, researchers can uncover patterns, trends, and relationships within their data. This section will provide an overview of dissertation data analysis, highlighting its significance in scholarly research.
The process of dissertation data analysis begins with the collection of relevant data. Researchers utilize different methods such as surveys, interviews, or experiments to gather the necessary information. Once the data is obtained, it needs to be carefully organized and prepared for analysis. This includes cleaning the data, checking for errors or inconsistencies, and transforming it into a suitable format for analysis.
The next step is to select appropriate data analysis techniques based on the research objectives and the nature of the data. Depending on the research design, researchers may use descriptive statistics to summarize and describe their data, or inferential statistics to make inferences or predictions about a larger population. Qualitative researchers, on the other hand, often employ techniques like thematic analysis or content analysis to explore patterns and themes within textual data.
In addition to statistical analysis, dissertation data analysis can also involve the use of specialized software tools. These tools provide researchers with the ability to perform complex calculations, generate visual representations of data, and conduct advanced statistical tests. By leveraging the power of technology, researchers are able to analyze large datasets more efficiently and effectively.
Overall, dissertation data analysis plays a pivotal role in extracting meaningful insights from research data. It enables researchers to draw evidence-based conclusions, validate hypotheses, and contribute to the existing body of knowledge in their field of study. By understanding and mastering the techniques of data analysis, scholars can unlock the potential of their research and make significant contributions to their respective disciplines.
Choosing the Right Data Analysis Methods
To successfully analyze the data for your dissertations and capstones, it is crucial to choose the appropriate data analysis methods. The selection of these methods will greatly impact the validity and reliability of your findings. Here are some key factors to consider when making this decision:
-
Research Questions and Objectives:
Begin by understanding the specific research questions and objectives of your study. This will help you determine the type of data analysis methods that align with your research goals. For instance, if you aim to explore the relationships between variables, statistical analysis techniques such as correlation or regression may be suitable. On the other hand, if your objective is to identify patterns or themes in qualitative data, qualitative analysis methods like thematic analysis or content analysis may be more appropriate. -
Data Type and Structure:
Consider the type of data you have collected and its structure. Are you dealing with numerical or categorical data? Is the data continuous or discrete? These characteristics will guide you in selecting the most suitable data analysis methods. Statistical techniques like t-tests or ANOVA are commonly used for numerical data, while chi-square tests are often used for categorical data. Additionally, if your data is hierarchical or has nested structures, multilevel analysis may be necessary. -
Available Resources and Expertise:
Assess your available resources, including time, software, and expertise. Some sophisticated data analysis methods may require specialized software or advanced statistical knowledge. If you have limited resources or expertise in a certain method, it may be wise to opt for simpler approaches that you can confidently execute. Consider seeking guidance from your advisor or collaborating with colleagues who have the necessary expertise in your chosen methods.
By carefully considering your research questions, data type, and available resources, you can choose the right data analysis methods for your dissertations and capstones. This will contribute to the overall quality and rigor of your study, enabling you to uncover meaningful insights and draw accurate conclusions.
Best Practices for Dissertation Data Analysis
In order to master dissertation data analysis, it is important to follow some best practices. These practices can help researchers effectively analyze their data and draw meaningful conclusions.
The first best practice is to ensure that the data collected is reliable and valid. This can be achieved by using appropriate research methods and data collection techniques. Researchers should carefully design their studies and implement rigorous data collection procedures to minimize errors and biases in the data.
Once the data is collected, organizing and cleaning it is crucial. Researchers should carefully structure their data sets, remove any irrelevant or duplicate data points, and handle missing values appropriately. This process ensures that the data is in a usable format for analysis and prevents any misleading results due to data quality issues.
Finally, selecting the right analysis methods is key to making sense of the data. Researchers should carefully consider their research questions and objectives, and choose the appropriate statistical techniques or qualitative analysis methods accordingly. This ensures that the analysis is aligned with the research goals and provides meaningful insights.
By following these best practices, researchers can effectively analyze their dissertation data and produce robust findings that contribute to their field of study.
Archives
- June 2025
- May 2025
- April 2025
- March 2025
- February 2025
- January 2025
- December 2024
- March 2024
- February 2024
- January 2024
- December 2023
- November 2023
- October 2023
- September 2023
- August 2023
- July 2023
- June 2023
- May 2023
- April 2023
- March 2023
- February 2023
- January 2023
- December 2022
- November 2022
- October 2022
- September 2022
- August 2022
- July 2022
- June 2022
- May 2022
- April 2022
- March 2022
- February 2022
- January 2022
Calendar
M | T | W | T | F | S | S |
---|---|---|---|---|---|---|
1 | ||||||
2 | 3 | 4 | 5 | 6 | 7 | 8 |
9 | 10 | 11 | 12 | 13 | 14 | 15 |
16 | 17 | 18 | 19 | 20 | 21 | 22 |
23 | 24 | 25 | 26 | 27 | 28 | 29 |
30 |